Tech
Gen AI Speeds Drug Discovery: Learn How It Works and See Real Examples
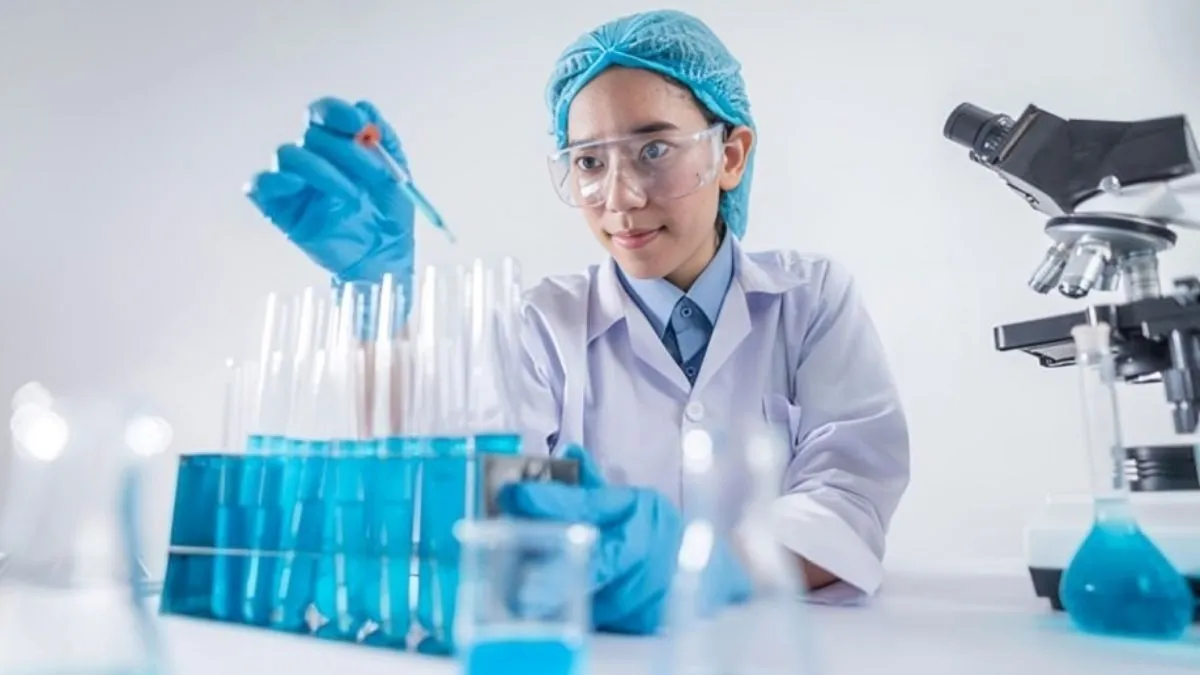
The pharmaceutical industry has always been at the forefront of technological innovation, but Generative Artificial Intelligence (Gen AI) is taking drug discovery to new heights. By leveraging the power of Gen AI, researchers can accelerate the discovery of new drugs, reduce development costs, and design novel molecules with higher efficacy. This breakthrough technology allows for the prediction of molecular interactions, optimization of drug candidates, and faster progression from concept to market. Pharma Gen AI is changing the drug development landscape, making it a crucial tool for the industry’s future.
Table of Contents
Understanding Generative AI in Drug Discovery
What is Generative AI?
Generative AI is a branch of artificial intelligence that focuses on creating new data, be it images, text, or, in the case of pharma, molecular structures. These models are trained using large datasets and can generate completely new compounds by learning from existing molecular data. By recognizing patterns and relationships between different molecules, generative AI in pharma can design new molecules that are more likely to have the desired therapeutic effects. This capability revolutionizes how researchers approach drug discovery, allowing them to bypass traditional trial-and-error methods and quickly pinpoint promising drug candidates.
How Does Gen AI Enhance Drug Discovery?
Gen AI enhances the drug discovery process in several transformative ways. Here’s how it works:
- Data Analysis: By processing vast datasets, AI models can identify potential drug targets much faster than traditional methods. This allows researchers to focus their efforts on the most promising molecules.
- Molecular Generation: Once a target is identified, AI can generate new molecular candidates by combining various chemical components in novel ways, leading to unique compounds with specific therapeutic properties.
- Predictive Modeling: AI can forecast how these molecules will behave in real-world biological systems, predicting their efficacy, safety, and potential side effects. This reduces the need for extensive preclinical testing and accelerates the path to clinical trials.
These capabilities streamline the drug development pipeline, significantly reducing time-to-market and optimizing the entire process.
Real-World Applications of Gen AI in Drug Discovery
Case Study 1: AlphaFold and Protein Structure Prediction
One of the most groundbreaking examples of Gen AI in pharma is AlphaFold, developed by DeepMind. This AI system predicts the 3D structure of proteins from their amino acid sequences, which has long been a challenge for researchers. Understanding protein structures is crucial in drug discovery, as it allows scientists to identify how proteins interact with other molecules. With AlphaFold, researchers can now quickly predict the structures of proteins, making it easier to identify new drug targets for diseases like Alzheimer’s and cancer. This has already accelerated the identification of potential drug candidates and is a major leap forward in drug development.
Case Study 2: AI-Designed Antibiotics
In the fight against antibiotic-resistant bacteria, Gen AI has proven its worth. Halicin, an AI-designed antibiotic, was discovered through AI’s ability to analyze and generate new molecular structures. Unlike traditional antibiotics, which target bacteria in one way, Halicin was designed to target bacteria through a completely novel mechanism, making it effective against strains that have become resistant to current treatments. This discovery highlights the ability of AI in pharma to design entirely new drugs that humans might not have thought of using traditional methods, offering a glimmer of hope in the fight against superbugs.
Case Study 3: Isomorphic Labs’ AI-Driven Drug Design
Isomorphic Labs, a company that leverages Generative AI in the pharmaceutical industry, has boldly revolutionized drug design. Using AI, the company can design drugs faster and more efficiently. Isomorphic Labs integrates Gen AI into every aspect of drug discovery, from identifying novel drug targets to designing and testing new molecules. This comprehensive approach allows for a more streamlined and efficient drug development process. Using AI to optimize and iterate on drug designs, Isomorphic Labs is paving the way for faster, more effective treatments.
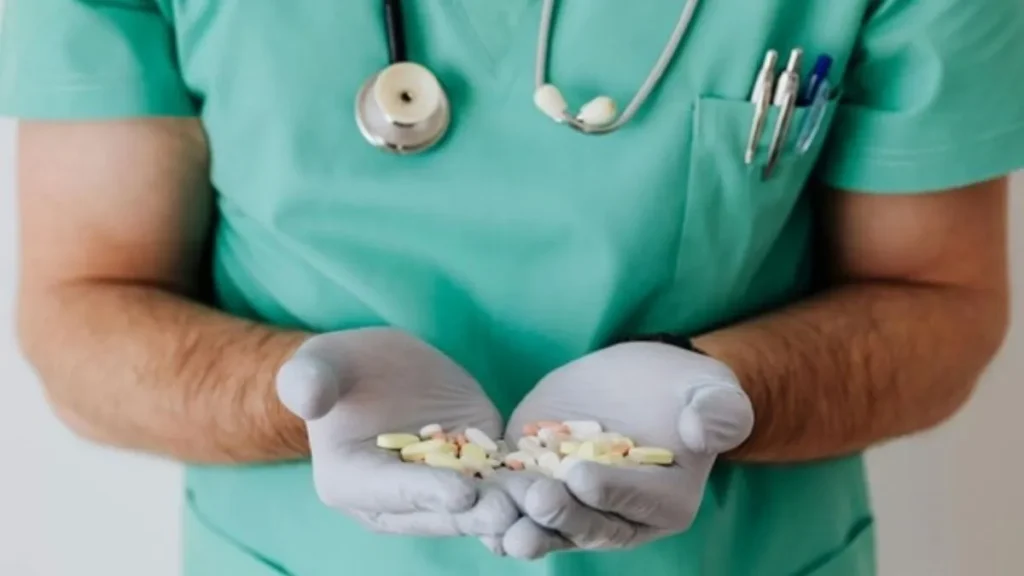
Benefits and Challenges of Integrating Gen AI in Drug Discovery
Benefits
The benefits of integrating Generative AI in pharma are clear:
- Accelerated Discovery: AI reduces the time required to identify promising drug candidates.
- Cost Efficiency: It lowers the financial barriers typically associated with drug development.
- Enhanced Precision: AI helps design molecules with greater specificity, improving the chances of developing targeted therapies.
These benefits help pharmaceutical companies develop drugs faster, reduce costs, and ultimately bring life-saving treatments to market more quickly.
Challenges
Despite its advantages, AI in pharma faces challenges:
- Data Quality: The effectiveness of AI is directly linked to the quality of data used. Poor data can lead to inaccurate predictions and ineffective drug candidates.
- Regulatory Hurdles: AI-generated drug candidates face regulatory challenges, as traditional methods are still the norm in drug approval processes.
- Integration with Traditional Methods: Merging AI-driven methods with conventional drug development processes can be complex, requiring collaboration and adaptation from human researchers and AI systems.
Future Outlook: The Role of Gen AI in the Next Era of Drug Discovery
As AI technology continues to evolve, the future of Gen AI in pharma looks incredibly promising. Emerging technologies like machine learning and artificial intelligence will enhance AI’s capabilities in drug discovery. We expect AI to play an even more significant role in creating personalized medicines, improving drug efficacy, and predicting treatment outcomes. With continuous advancements, AI could design more effective, safer, and tailored drugs to individual genetic profiles. This would mark a significant shift towards precision medicine in the healthcare industry.
Conclusion
Gen AI is changing drug discovery by accelerating the development of new drugs and optimizing every step of the process. From AlphaFold’s protein structure predictions to the creation of AI-designed antibiotics, real-world examples show the immense potential of Gen AI in pharma. While challenges remain, the future is bright for AI-driven drug discovery. We can look forward to more efficient, cost-effective, and targeted treatments as technology advances. Integrating Gen AI in pharma is a step towards a more innovative and sustainable future for the healthcare industry.
-
GENERAL2 months ago
Uncovering the World of кинокрадко: The Dark Side of Film Piracy
-
GENERAL4 weeks ago
Unveiling the Art of преводсч: How Translators Bridge Language Barriers
-
YOGA1 year ago
4 Person Yoga Poses for Beginners
-
GENERAL2 months ago
The Journey of iamnobody89757: From Anonymous User to Internet Sensation